Register Now
Join the year’s premier education event
on open data architectures for data practitioners
Lineup
- 2 tracks of expert-led content
- Panels
- Workshops
- Technical sessions
Topics
- Open data architectures for AI & ML
- Analytics
- Data engineering
- Data lakehouses
- Data transformations
- Query engines
- Stream processing
- and more!
Audience
- Data engineers
- Data architects
- Analytics engineers
- Big data engineers
- Cloud data engineers
- DataOps
- Data pipeline engineers
Speakers

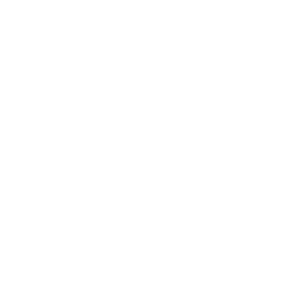
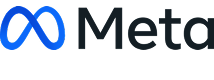
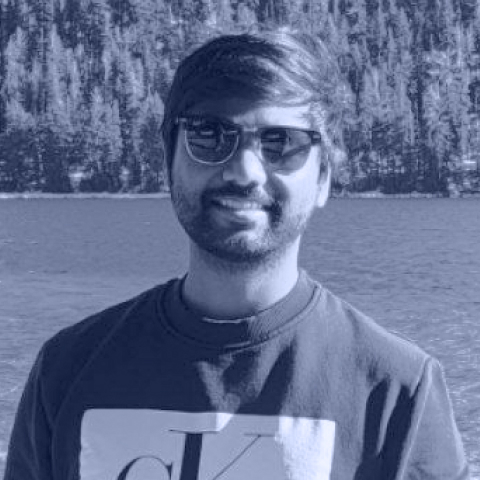
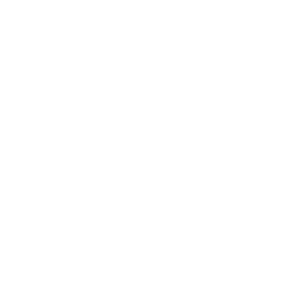
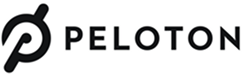
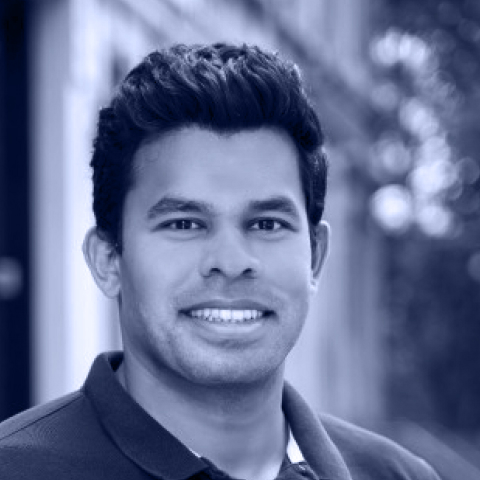
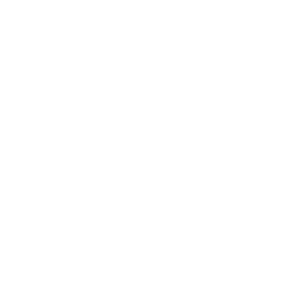
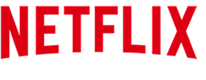
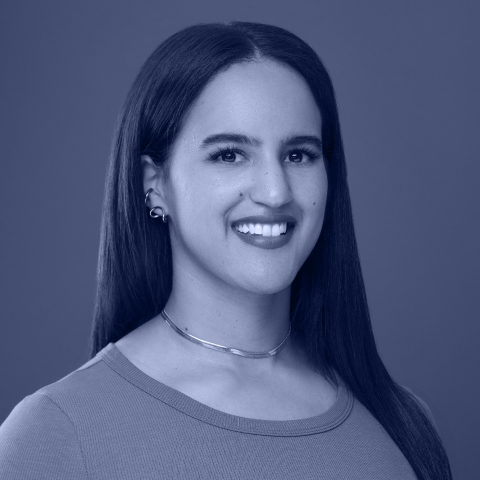
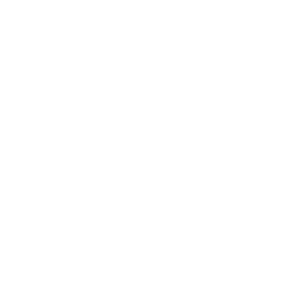
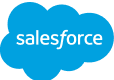
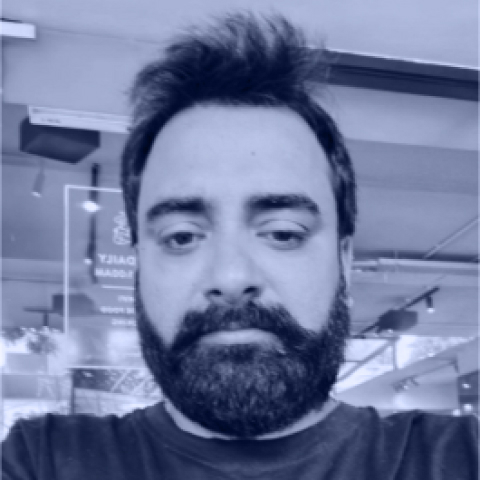
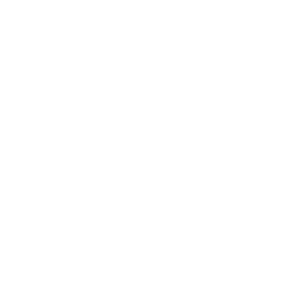
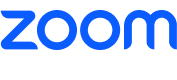

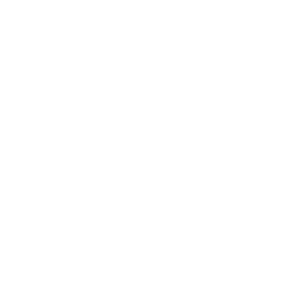
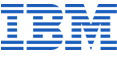
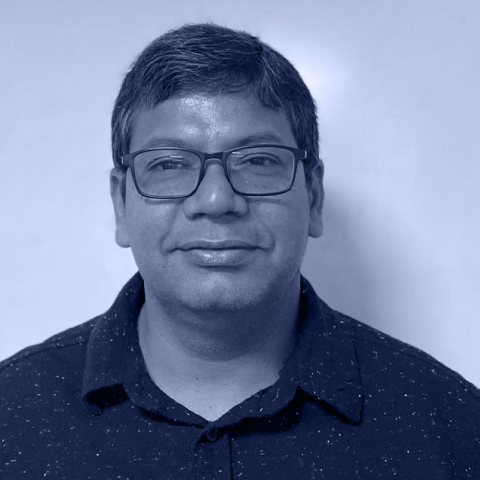
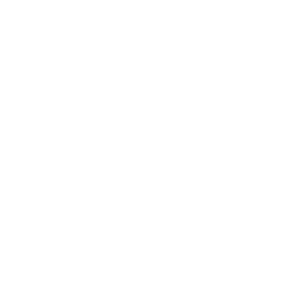
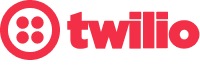
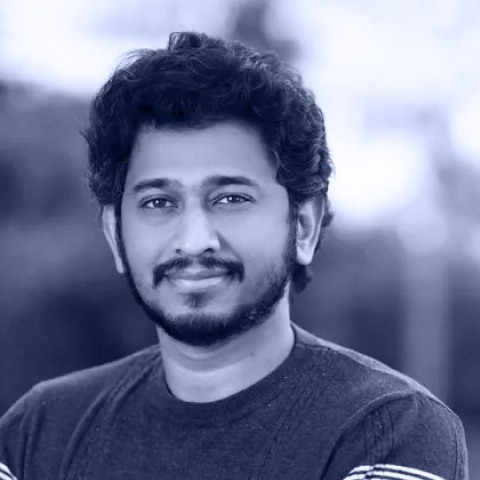
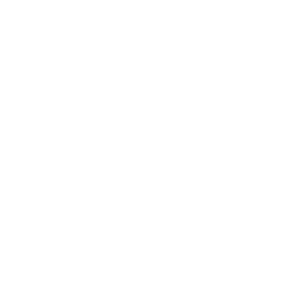
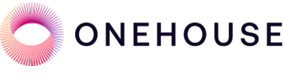

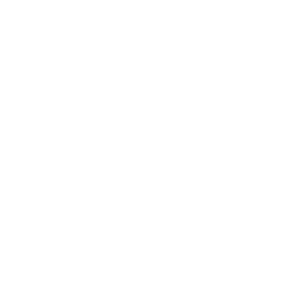
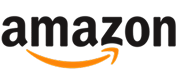
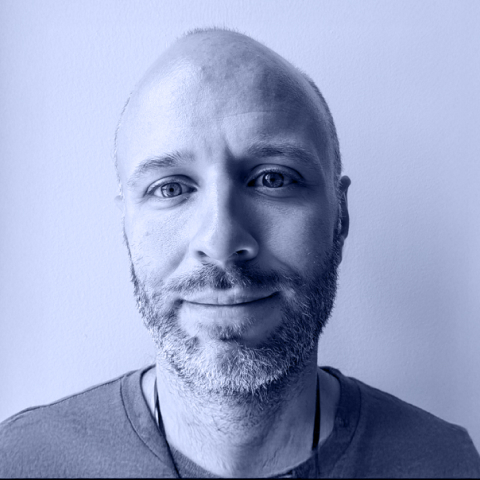
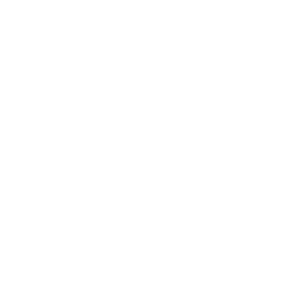
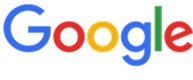
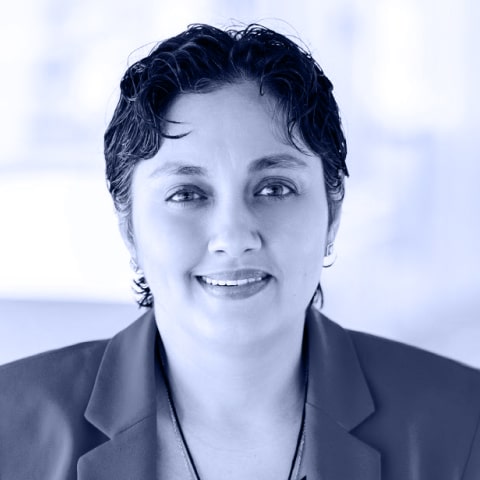
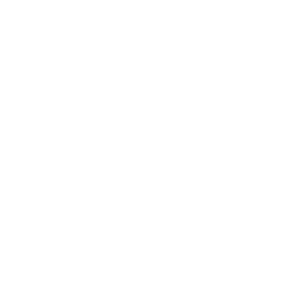
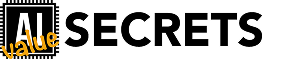

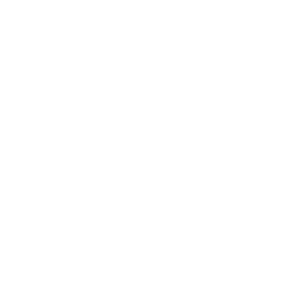
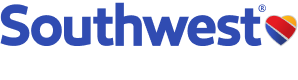
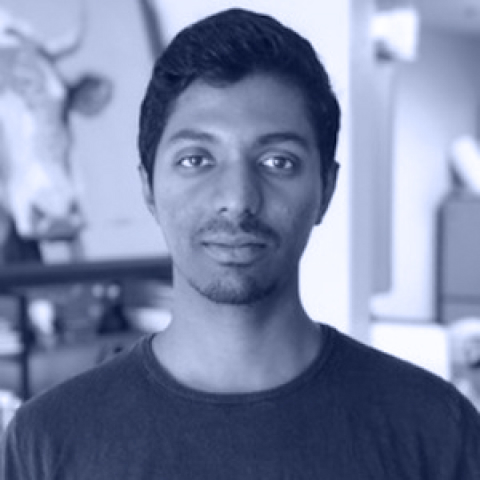
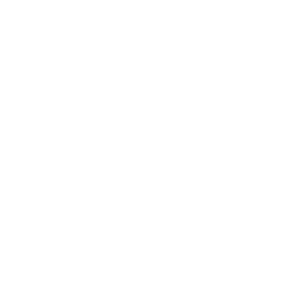
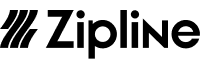
.jpg)
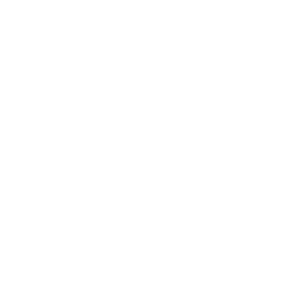
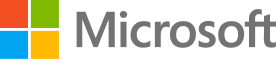

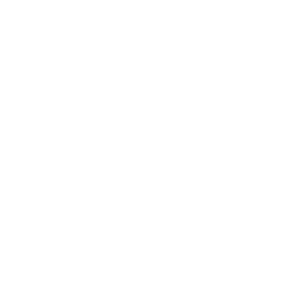
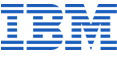
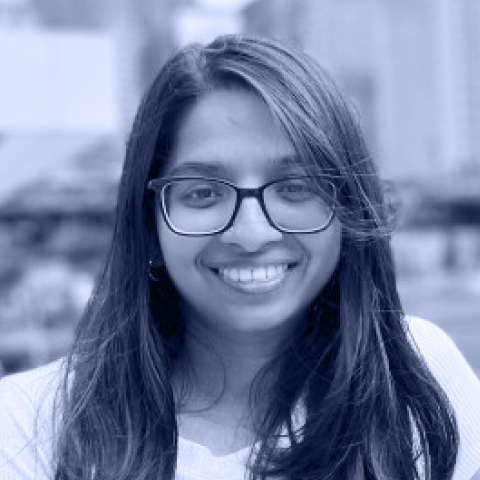
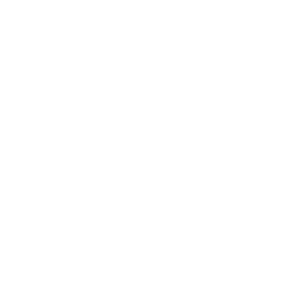

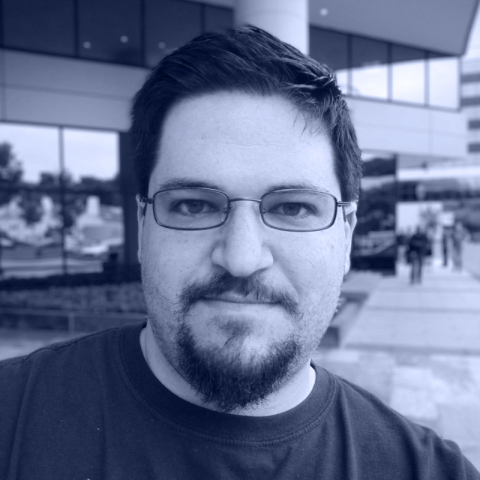
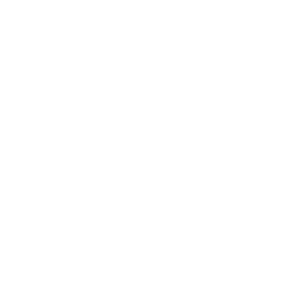


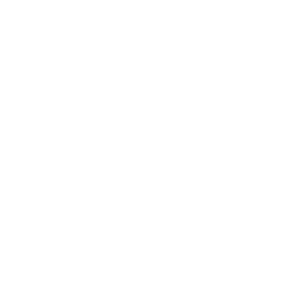
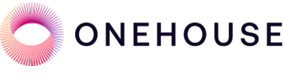
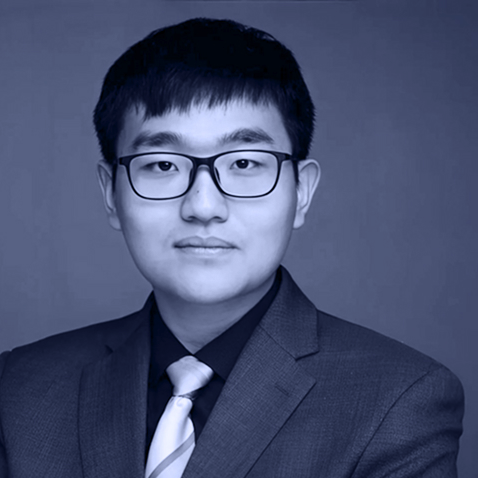
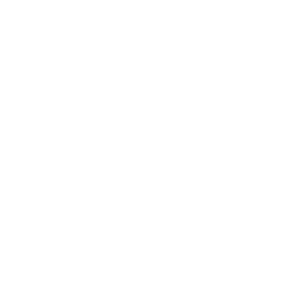

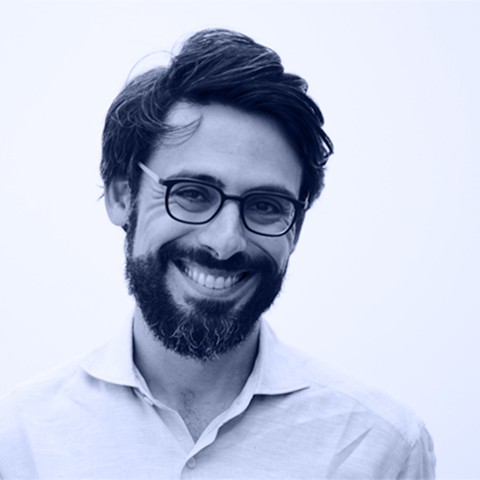
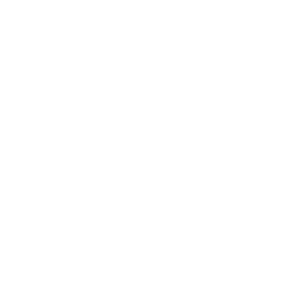
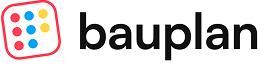
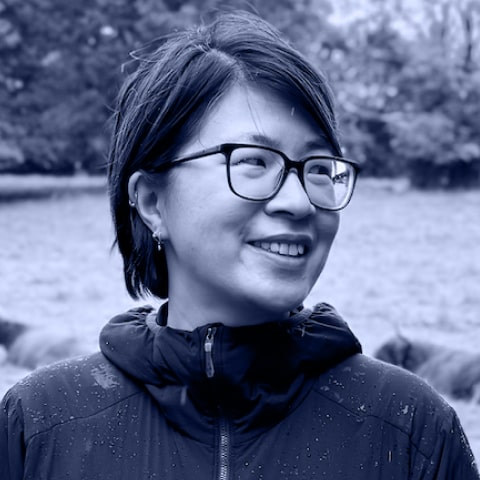
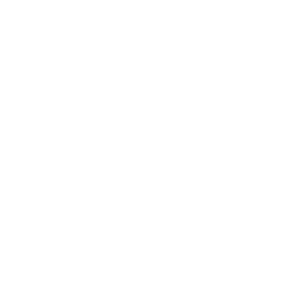
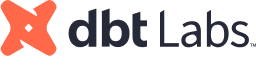

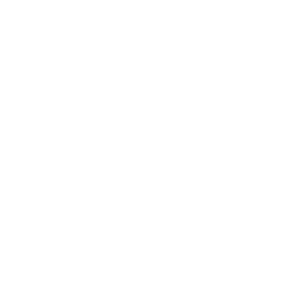
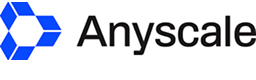

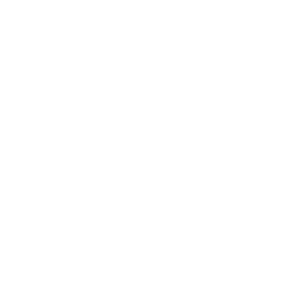
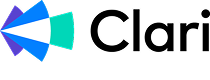
Keynotes
Two very big things happened in recent years that completely transformed the data landscape:
- Pre-trained AI models: These models have democratized AI, enabling software engineers to integrate advanced capabilities into applications with simple API calls and without extensive machine learning expertise.
- Lakehouses: Open formats over object storage bring together the flexibility of data lakes and the data management strengths of data warehouses, offering a more streamlined and scalable approach to data management.
And yet, most data platforms remain difficult to digest for traditional software developers.
This talk introduces "Data as Software," a practical approach to data engineering and AI that leverages the lakehouse architecture to simplify data platforms for developers. By leveraging serverless functions as a runtime and Git-based workflows in the data catalog, we can build systems that makes it exponentially simpler for data developers to apply familiar software engineering concepts to data, such as modular, reusable code, automated testing (TDD), continuous integration (CI/CD), and version control.
Two very big things happened in recent years that completely transformed the data landscape:
- Pre-trained AI models: These models have democratized AI, enabling software engineers to integrate advanced capabilities into applications with simple API calls and without extensive machine learning expertise.
- Lakehouses: Open formats over object storage bring together the flexibility of data lakes and the data management strengths of data warehouses, offering a more streamlined and scalable approach to data management.
And yet, most data platforms remain difficult to digest for traditional software developers.
This talk introduces "Data as Software," a practical approach to data engineering and AI that leverages the lakehouse architecture to simplify data platforms for developers. By leveraging serverless functions as a runtime and Git-based workflows in the data catalog, we can build systems that makes it exponentially simpler for data developers to apply familiar software engineering concepts to data, such as modular, reusable code, automated testing (TDD), continuous integration (CI/CD), and version control.

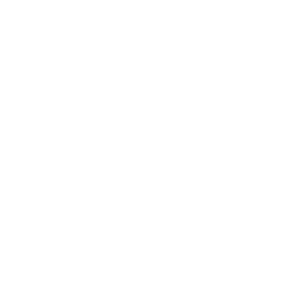
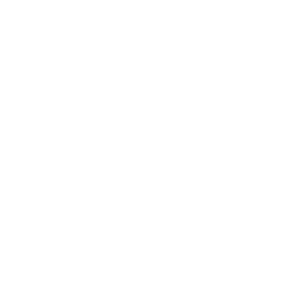
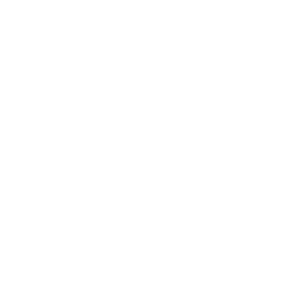
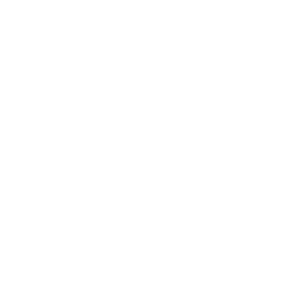
In today’s rush to adopt AI, many organizations overlook a critical truth: value doesn’t come from AI alone—it comes from the powerful combination of software engineering, data engineering, and AI/ML engineering. In this fast-paced, 15-minute talk, Nisha Paliwal draws on 25+ years of experience in banking and tech to unpack the "Trifacta" that fuels real transformation.
From legacy systems to self-learning platforms, she’ll share stories, stats, and insights on how this triad—when integrated—enables banks to move faster, deliver smarter experiences, and generate measurable impact.
Whether you're a technologist, leader, or change agent, you’ll walk away with a fresh lens on cross-functional collaboration, and practical ways to break silos, build trust, and unlock innovation at scale.
In today’s rush to adopt AI, many organizations overlook a critical truth: value doesn’t come from AI alone—it comes from the powerful combination of software engineering, data engineering, and AI/ML engineering. In this fast-paced, 15-minute talk, Nisha Paliwal draws on 25+ years of experience in banking and tech to unpack the "Trifacta" that fuels real transformation.
From legacy systems to self-learning platforms, she’ll share stories, stats, and insights on how this triad—when integrated—enables banks to move faster, deliver smarter experiences, and generate measurable impact.
Whether you're a technologist, leader, or change agent, you’ll walk away with a fresh lens on cross-functional collaboration, and practical ways to break silos, build trust, and unlock innovation at scale.

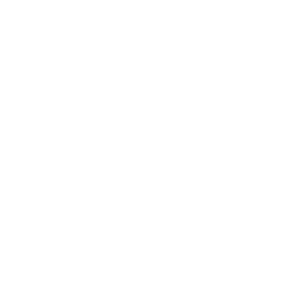
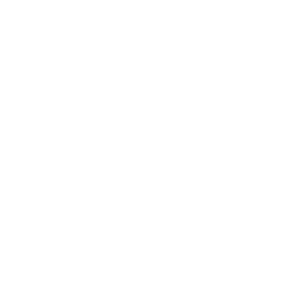
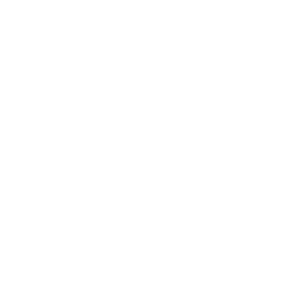
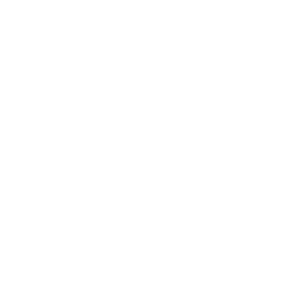
Apache Gluten™ (incubating) is an emerging open-source project in the Apache software ecosystem. It's designed to enhance the performance and scalability of data processing frameworks such as Apache Spark™. By leveraging cutting-edge technologies such as vectorized execution, columnar data formats, and advanced memory management techniques, Apache Gluten aims to deliver significant improvements in data processing speed and efficiency.
The primary goal of Apache Gluten is to address the ever-growing demand for real-time data analytics and large-scale data processing. It achieves this by optimizing the execution of complex data processing tasks and reducing the overall resource consumption. As a result, organizations can process massive datasets more quickly and cost-effectively, enabling them to gain valuable insights and make data-driven decisions faster than ever before.
Apache Gluten™ (incubating) is an emerging open-source project in the Apache software ecosystem. It's designed to enhance the performance and scalability of data processing frameworks such as Apache Spark™. By leveraging cutting-edge technologies such as vectorized execution, columnar data formats, and advanced memory management techniques, Apache Gluten aims to deliver significant improvements in data processing speed and efficiency.
The primary goal of Apache Gluten is to address the ever-growing demand for real-time data analytics and large-scale data processing. It achieves this by optimizing the execution of complex data processing tasks and reducing the overall resource consumption. As a result, organizations can process massive datasets more quickly and cost-effectively, enabling them to gain valuable insights and make data-driven decisions faster than ever before.
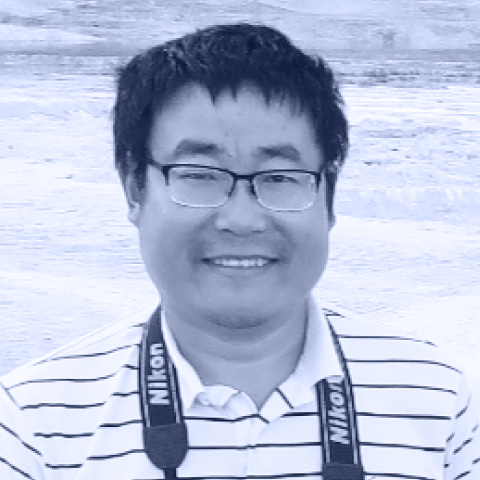
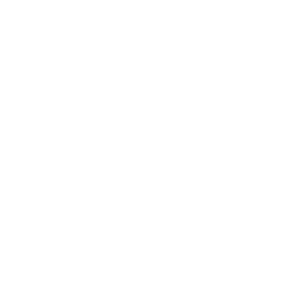
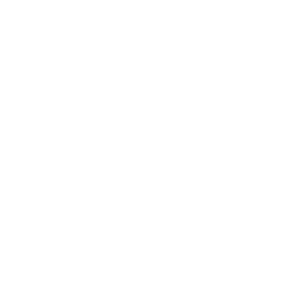
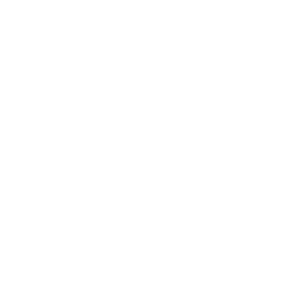
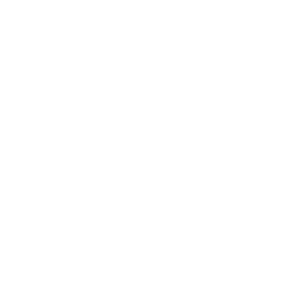
This talk delves into the revolutionary potential of AI agents, powered by generative AI and large language models (LLMs), in transforming ETL (Extract, Transform, Load) and ELT (Extract, Load, Transform) processes.
With an emphasis on automating code generation, streamlining workflows, and enhancing data quality, this session explores how AI-driven solutions are reshaping the landscape of data engineering. Attendees will learn how these intelligent agents can reduce manual coding, eliminate errors, improve operational efficiency, and meet compliance requirements, all while accelerating development timelines.
We will also cover key use cases where AI agents facilitate real-time data transformation, ensure data governance, and promote seamless deployment across cloud environments, giving businesses a competitive edge in today's data-driven world.
This talk delves into the revolutionary potential of AI agents, powered by generative AI and large language models (LLMs), in transforming ETL (Extract, Transform, Load) and ELT (Extract, Load, Transform) processes.
With an emphasis on automating code generation, streamlining workflows, and enhancing data quality, this session explores how AI-driven solutions are reshaping the landscape of data engineering. Attendees will learn how these intelligent agents can reduce manual coding, eliminate errors, improve operational efficiency, and meet compliance requirements, all while accelerating development timelines.
We will also cover key use cases where AI agents facilitate real-time data transformation, ensure data governance, and promote seamless deployment across cloud environments, giving businesses a competitive edge in today's data-driven world.

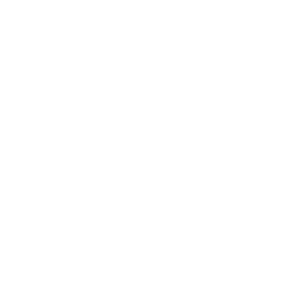
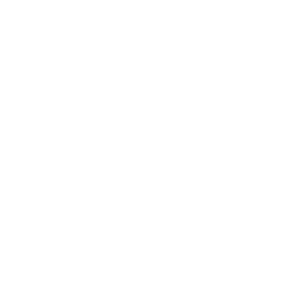
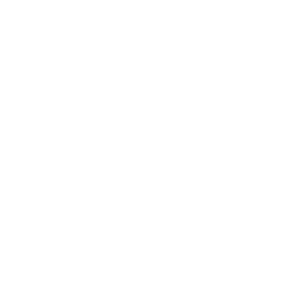
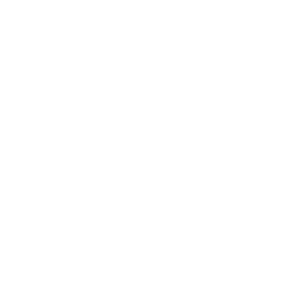
The data lakehouse architecture has made big waves in recent years. But there are so many considerations. Which table formats should you start with? What file formats are the most performant? With which data catalogs and query engines do you need to integrate? To be honest, it can become a bit overwhelming.
But what data engineer doesn't like a good technical challenge? This is where it sometimes becomes a philosophical decision of build vs buy.
In this presentation, Onehouse VP of Product Kyle Weller will break down the pros and cons he has seen over nearly a decade of helping organizations implement their own data lakehouses and building the Universal Data Lakehouse at Onehouse. You'll learn about:
- The strengths of open table formats such as Apache Hudi™, Apache Iceberg™ and Delta Lake
- Interoperability via abstraction layers such as Apache XTable™ (incubating)
- Lakehouse optimizations for cost and performance via Apache Spark™-based runtimes
The data lakehouse architecture has made big waves in recent years. But there are so many considerations. Which table formats should you start with? What file formats are the most performant? With which data catalogs and query engines do you need to integrate? To be honest, it can become a bit overwhelming.
But what data engineer doesn't like a good technical challenge? This is where it sometimes becomes a philosophical decision of build vs buy.
In this presentation, Onehouse VP of Product Kyle Weller will break down the pros and cons he has seen over nearly a decade of helping organizations implement their own data lakehouses and building the Universal Data Lakehouse at Onehouse. You'll learn about:
- The strengths of open table formats such as Apache Hudi™, Apache Iceberg™ and Delta Lake
- Interoperability via abstraction layers such as Apache XTable™ (incubating)
- Lakehouse optimizations for cost and performance via Apache Spark™-based runtimes

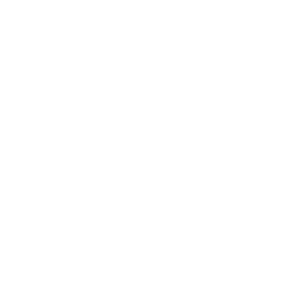
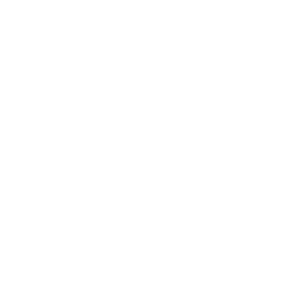
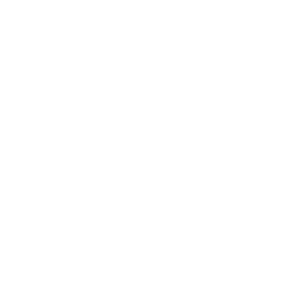
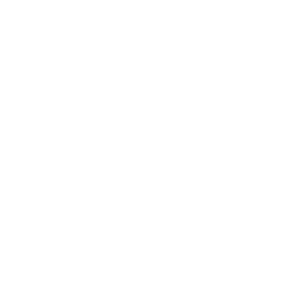
Understanding and improving unit-level profitability at Amazon's scale is a massive challenge, one that requires flexibility, precision, and operational efficiency. It's not only about the massive amount of data we ingest and produce, but also the need to support our evergrowing businesses within Amazon. In this talk, we'll walk through how we built a scalable, configuration-driven platform called Nexus, and how Apache Hudi™ became the cornerstone of its data lake architecture.
Understanding and improving unit-level profitability at Amazon's scale is a massive challenge, one that requires flexibility, precision, and operational efficiency. It's not only about the massive amount of data we ingest and produce, but also the need to support our evergrowing businesses within Amazon. In this talk, we'll walk through how we built a scalable, configuration-driven platform called Nexus, and how Apache Hudi™ became the cornerstone of its data lake architecture.
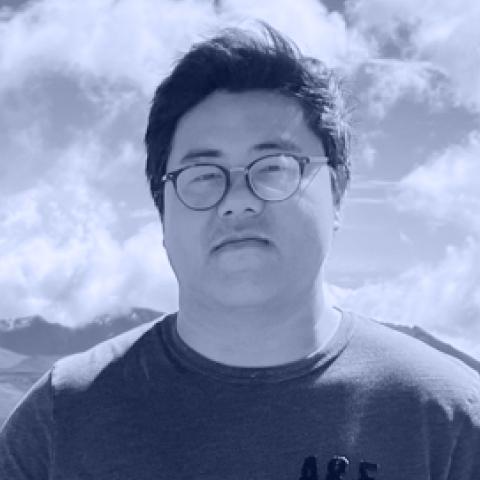
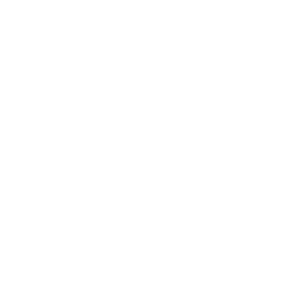
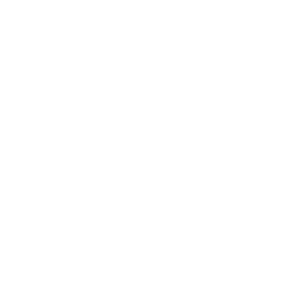
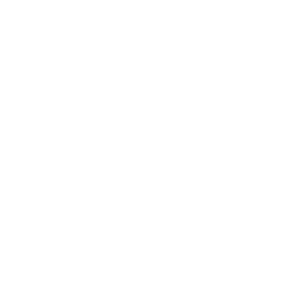
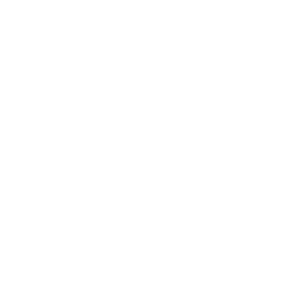
OneLake eliminates pervasive and chaotic data silos created by developers configuring their own isolated storage. OneLake provides a single, unified storage system for all developers. Unifying data across an organization and clouds becomes trivial. With the OneLake data hub, users can easily explore and discover data to reuse, manage or gain insights. With business domains, different business units can work independently in a data mesh pattern, without the overhead of maintaining separate data stores.
OneLake eliminates pervasive and chaotic data silos created by developers configuring their own isolated storage. OneLake provides a single, unified storage system for all developers. Unifying data across an organization and clouds becomes trivial. With the OneLake data hub, users can easily explore and discover data to reuse, manage or gain insights. With business domains, different business units can work independently in a data mesh pattern, without the overhead of maintaining separate data stores.
.jpeg)
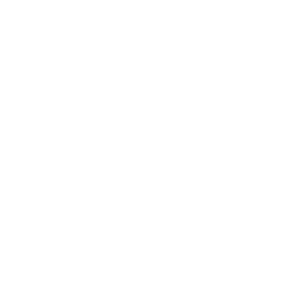
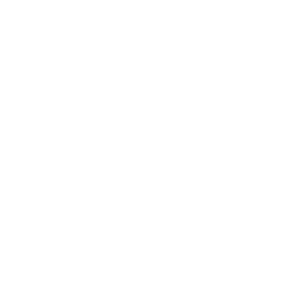
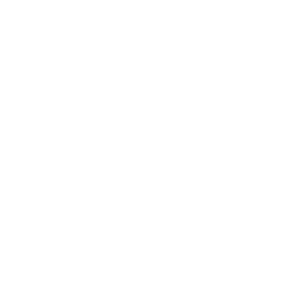
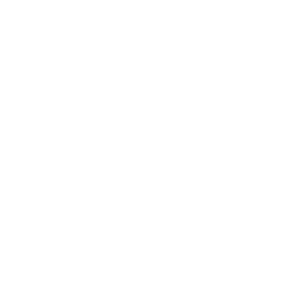
AI agents need more than just language - they need to act. This talk introduces trajectory data - an emerging class of data used to training LLM agents. These are sequences of observations, actions, and outcomes that drive agent learning. Whether you're training agents or building the data pipelines behind them, this is your guide to the data powering the next generation of AI agents.
AI agents need more than just language - they need to act. This talk introduces trajectory data - an emerging class of data used to training LLM agents. These are sequences of observations, actions, and outcomes that drive agent learning. Whether you're training agents or building the data pipelines behind them, this is your guide to the data powering the next generation of AI agents.

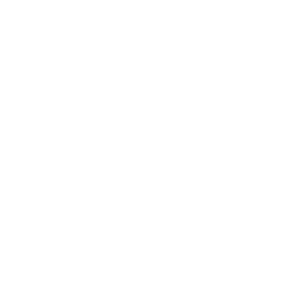
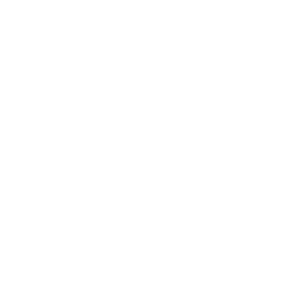
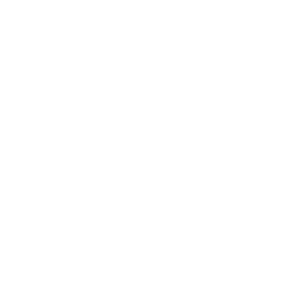
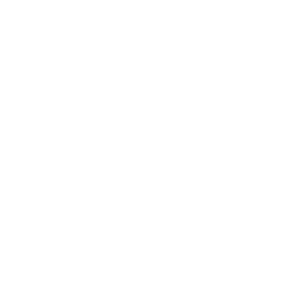
In the coming years, use of unstructured and multi-modal data for AI workloads will grow exponentially. This talk will focus on how Ray Data effectively scales data processing for these modalities across heterogeneous architectures and is positioned to become a key component of future AI platforms
In the coming years, use of unstructured and multi-modal data for AI workloads will grow exponentially. This talk will focus on how Ray Data effectively scales data processing for these modalities across heterogeneous architectures and is positioned to become a key component of future AI platforms
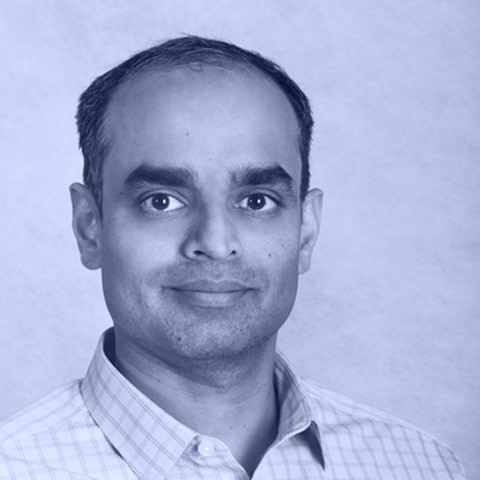
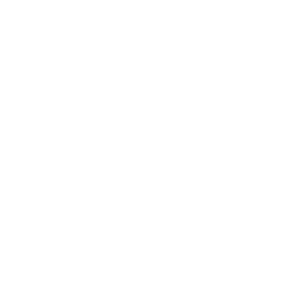
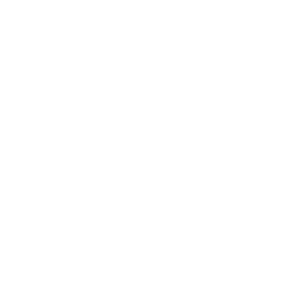
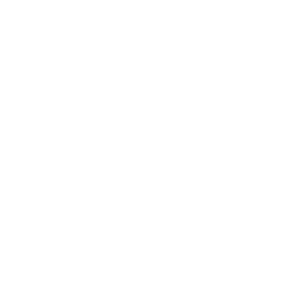
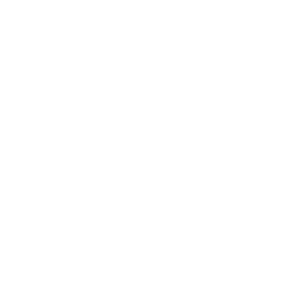
In this talk, I will go over some of the implementation details of how we built a Data Lake for Clari by using a federated query engine built on Trino & Airflow while using Iceberg as the data storage format.
Clari is an Enterprise Revenue Orchestration Platform helping customers run their revenue cadences and helping sales teams close deals more efficiently. Being an enterprise company, we have strict legal requirements of following data governance policies.
I will cover how to design a scalable architecture for building data ingestion pipelines for bringing together data from various sources for the purposes of AI and ML.
I will also cover some of the use cases that have been unlocked in the company with respect to building Agentic Frameworks with the development of this data lake.
In this talk, I will go over some of the implementation details of how we built a Data Lake for Clari by using a federated query engine built on Trino & Airflow while using Iceberg as the data storage format.
Clari is an Enterprise Revenue Orchestration Platform helping customers run their revenue cadences and helping sales teams close deals more efficiently. Being an enterprise company, we have strict legal requirements of following data governance policies.
I will cover how to design a scalable architecture for building data ingestion pipelines for bringing together data from various sources for the purposes of AI and ML.
I will also cover some of the use cases that have been unlocked in the company with respect to building Agentic Frameworks with the development of this data lake.

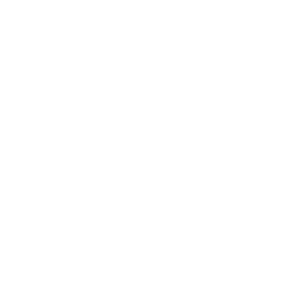
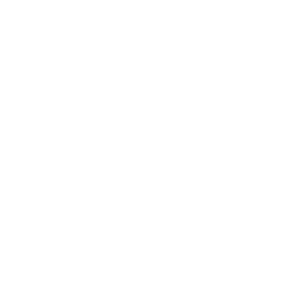
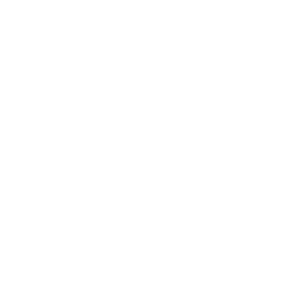
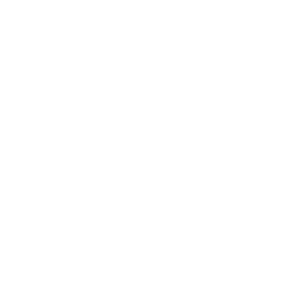
As the adoption of LLMs continues to expand, awareness of the risks associated with them is also increasing. It is essential to manage these risks effectively amidst the ongoing hype, technological optimism, and fear-driven narratives. This presentation will explore how to address vulnerabilities that may emerge. Our focus will extend beyond simply securing interactions with the models, emphasizing the critical role of surrounding infrastructure and monitoring practices.
The talk will introduce a structured framework for developing "system-level secure" AI deployments from the ground up. This framework covers pre-deployment risks (such as poisoned models), deployment risks (including model deserialization), and online attack vectors (such as prompt injection). Drawing on two years of experience deploying AI systems in sensitive environments with strict privacy and security requirements, the talk will provide actionable strategies to help organizations build secure, resilient applications using open-source LLMs. Attendees will gain practical insights into strengthening both AI models and the supporting infrastructure, equipping them to develop robust AI solutions in an increasingly complex threat environment.
As the adoption of LLMs continues to expand, awareness of the risks associated with them is also increasing. It is essential to manage these risks effectively amidst the ongoing hype, technological optimism, and fear-driven narratives. This presentation will explore how to address vulnerabilities that may emerge. Our focus will extend beyond simply securing interactions with the models, emphasizing the critical role of surrounding infrastructure and monitoring practices.
The talk will introduce a structured framework for developing "system-level secure" AI deployments from the ground up. This framework covers pre-deployment risks (such as poisoned models), deployment risks (including model deserialization), and online attack vectors (such as prompt injection). Drawing on two years of experience deploying AI systems in sensitive environments with strict privacy and security requirements, the talk will provide actionable strategies to help organizations build secure, resilient applications using open-source LLMs. Attendees will gain practical insights into strengthening both AI models and the supporting infrastructure, equipping them to develop robust AI solutions in an increasingly complex threat environment.
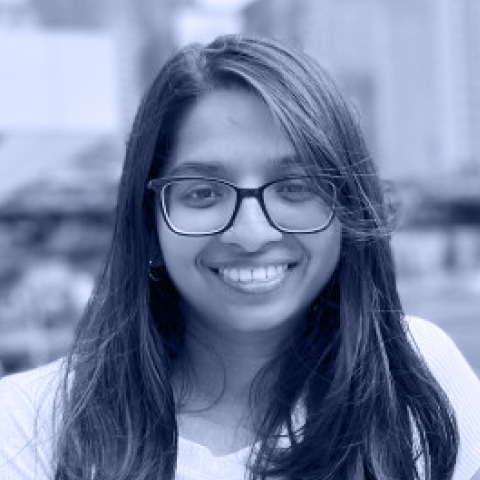
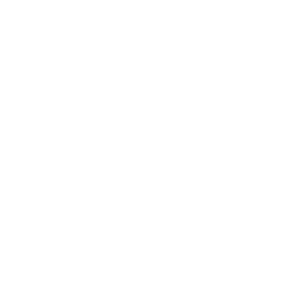
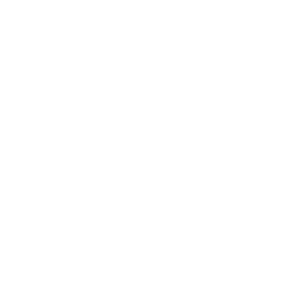
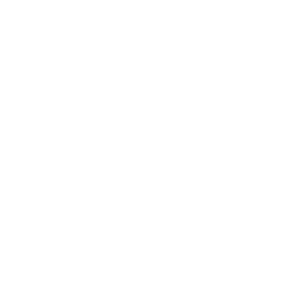
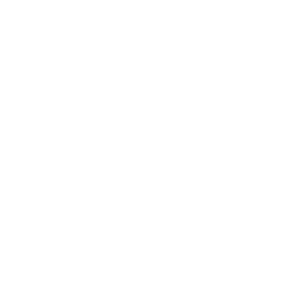
Lorem Ipsum Dolor Sit Amet
AI agents need more than just language - they need to act. This talk introduces trajectory data - an emerging class of data used to training LLM agents. These are sequences of observations, actions, and outcomes that drive agent learning. Whether you're training agents or building the data pipelines behind them, this is your guide to the data powering the next generation of AI agents.

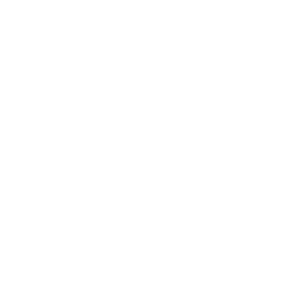
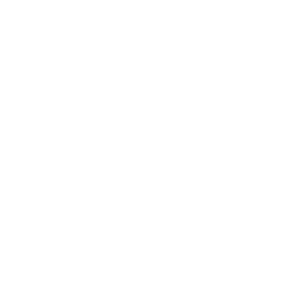
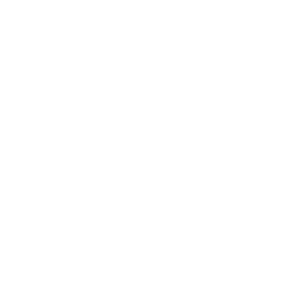
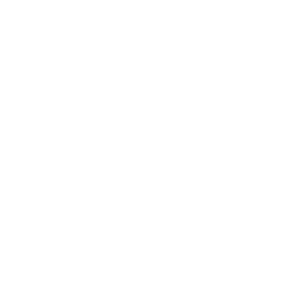
OneLake eliminates pervasive and chaotic data silos created by developers configuring their own isolated storage. OneLake provides a single, unified storage system for all developers. Unifying data across an organization and clouds becomes trivial. With the OneLake data hub, users can easily explore and discover data to reuse, manage or gain insights. With business domains, different business units can work independently in a data mesh pattern, without the overhead of maintaining separate data stores.
.jpeg)
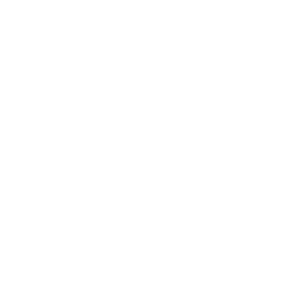
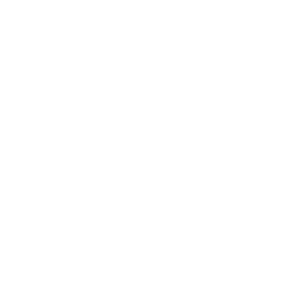
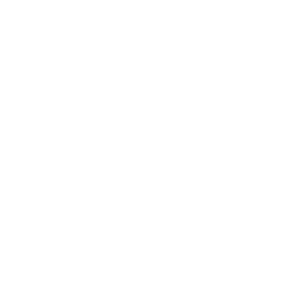
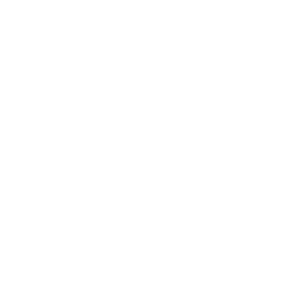
The data lakehouse architecture has made big waves in recent years. But there are so many considerations. Which table formats should you start with? What file formats are the most performant? With which data catalogs and query engines do you need to integrate? To be honest, it can become a bit overwhelming.
But what data engineer doesn't like a good technical challenge? This is where it sometimes becomes a philosophical decision of build vs buy.
In this presentation, Onehouse VP of Product Kyle Weller will break down the pros and cons he has seen over nearly a decade of helping organizations implement their own data lakehouses and building the Universal Data Lakehouse at Onehouse. You'll learn about:
- The strengths of open table formats such as Apache Hudi™, Apache Iceberg™ and Delta Lake
- Interoperability via abstraction layers such as Apache XTable™ (incubating)
- Lakehouse optimizations for cost and performance via Apache Spark™-based runtimes

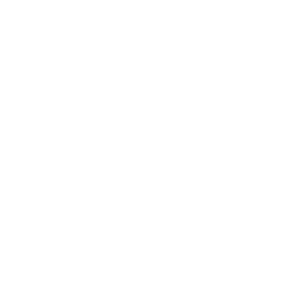
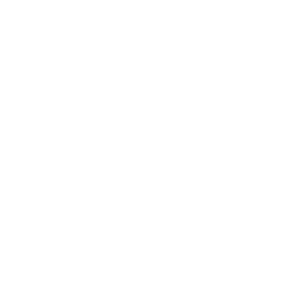
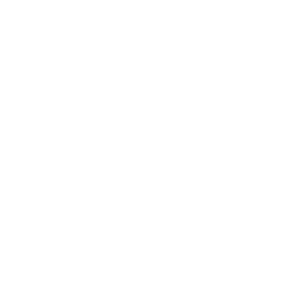
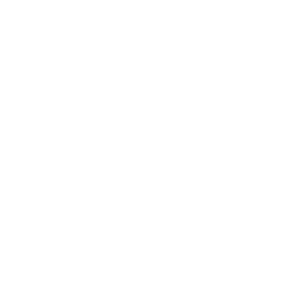
In this talk, I will go over some of the implementation details of how we built a Data Lake for Clari by using a federated query engine built on Trino & Airflow while using Iceberg as the data storage format.
Clari is an Enterprise Revenue Orchestration Platform helping customers run their revenue cadences and helping sales teams close deals more efficiently. Being an enterprise company, we have strict legal requirements of following data governance policies.
I will cover how to design a scalable architecture for building data ingestion pipelines for bringing together data from various sources for the purposes of AI and ML.
I will also cover some of the use cases that have been unlocked in the company with respect to building Agentic Frameworks with the development of this data lake.

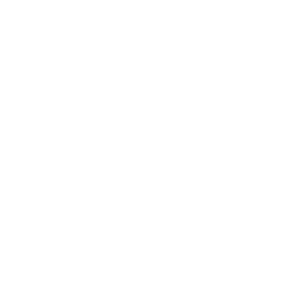
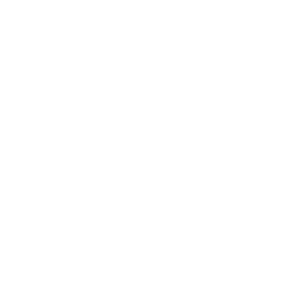
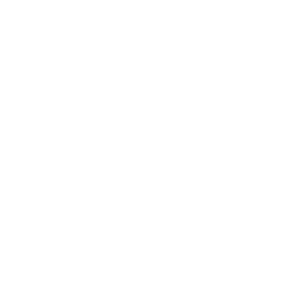
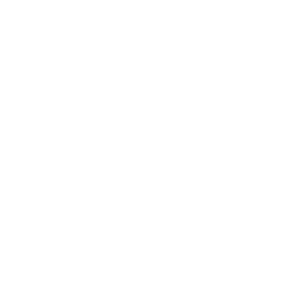
Understanding and improving unit-level profitability at Amazon's scale is a massive challenge, one that requires flexibility, precision, and operational efficiency. It's not only about the massive amount of data we ingest and produce, but also the need to support our evergrowing businesses within Amazon. In this talk, we'll walk through how we built a scalable, configuration-driven platform called Nexus, and how Apache Hudi™ became the cornerstone of its data lake architecture.
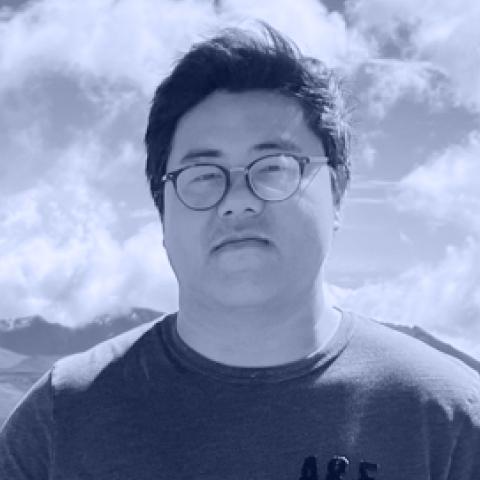
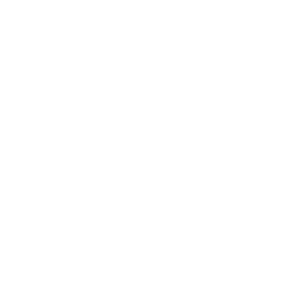
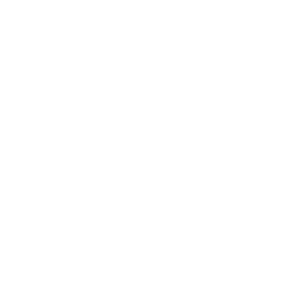
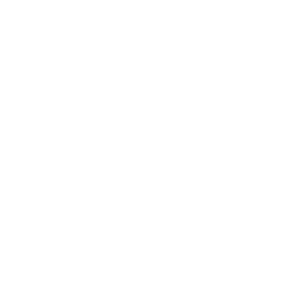
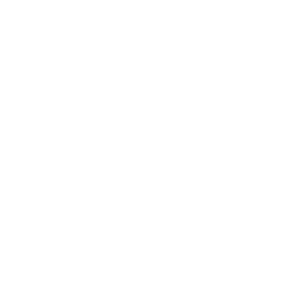
In the coming years, use of unstructured and multi-modal data for AI workloads will grow exponentially. This talk will focus on how Ray Data effectively scales data processing for these modalities across heterogeneous architectures and is positioned to become a key component of future AI platforms
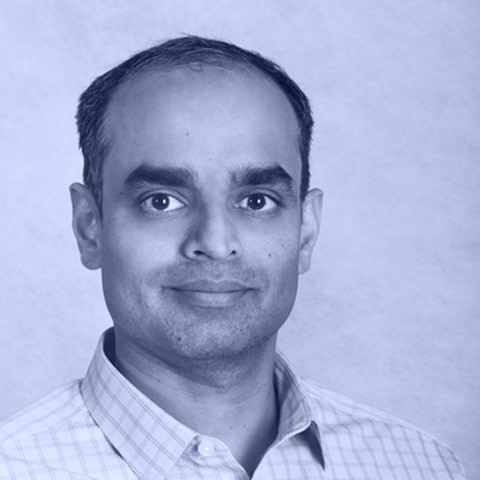
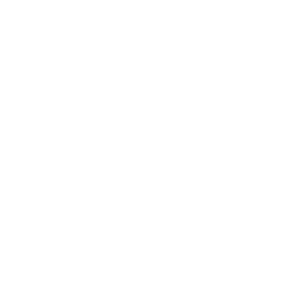
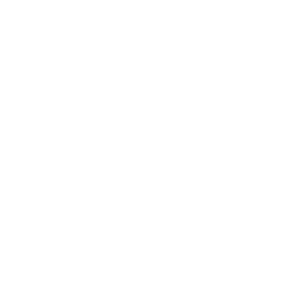
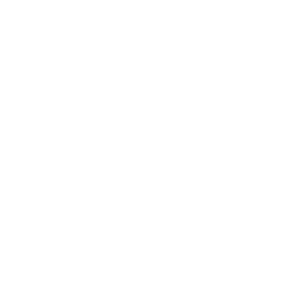
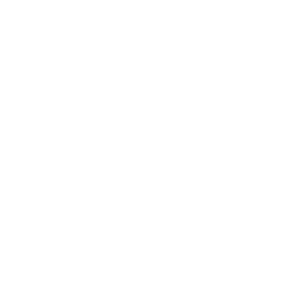
Apache Gluten™ (incubating) is an emerging open-source project in the Apache software ecosystem. It's designed to enhance the performance and scalability of data processing frameworks such as Apache Spark™. By leveraging cutting-edge technologies such as vectorized execution, columnar data formats, and advanced memory management techniques, Apache Gluten aims to deliver significant improvements in data processing speed and efficiency.
The primary goal of Apache Gluten is to address the ever-growing demand for real-time data analytics and large-scale data processing. It achieves this by optimizing the execution of complex data processing tasks and reducing the overall resource consumption. As a result, organizations can process massive datasets more quickly and cost-effectively, enabling them to gain valuable insights and make data-driven decisions faster than ever before.
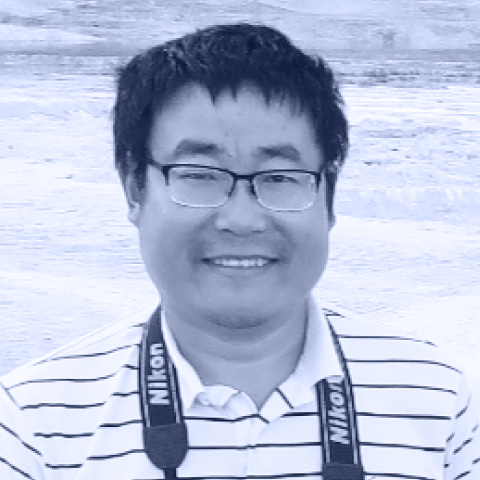
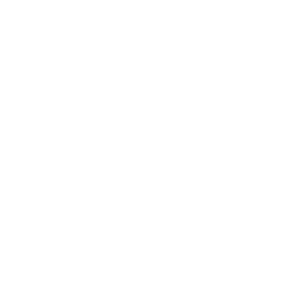
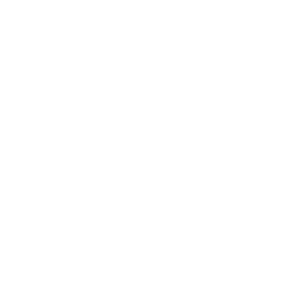
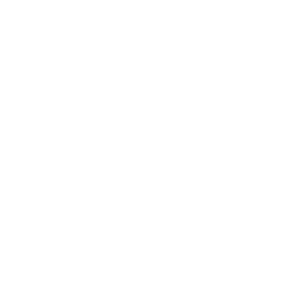
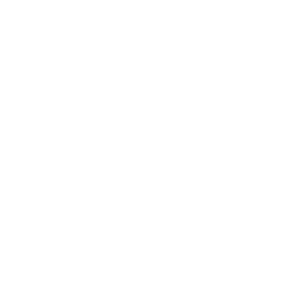
As the adoption of LLMs continues to expand, awareness of the risks associated with them is also increasing. It is essential to manage these risks effectively amidst the ongoing hype, technological optimism, and fear-driven narratives. This presentation will explore how to address vulnerabilities that may emerge. Our focus will extend beyond simply securing interactions with the models, emphasizing the critical role of surrounding infrastructure and monitoring practices.
The talk will introduce a structured framework for developing "system-level secure" AI deployments from the ground up. This framework covers pre-deployment risks (such as poisoned models), deployment risks (including model deserialization), and online attack vectors (such as prompt injection). Drawing on two years of experience deploying AI systems in sensitive environments with strict privacy and security requirements, the talk will provide actionable strategies to help organizations build secure, resilient applications using open-source LLMs. Attendees will gain practical insights into strengthening both AI models and the supporting infrastructure, equipping them to develop robust AI solutions in an increasingly complex threat environment.
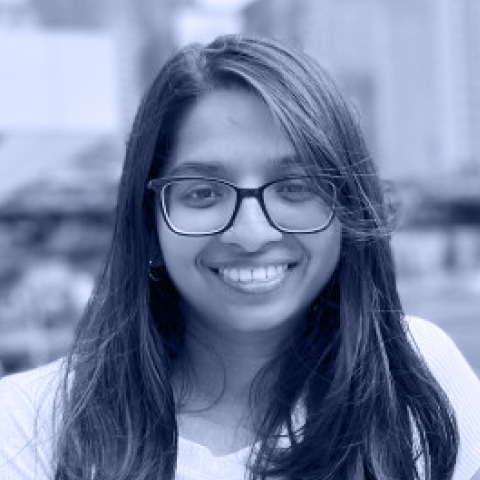
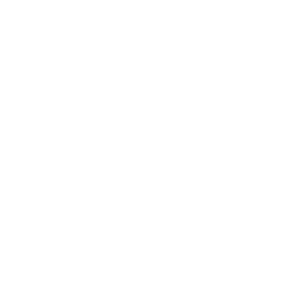
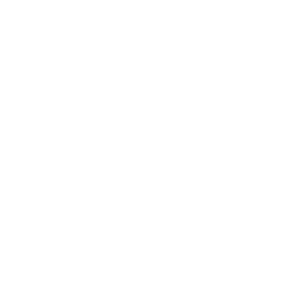
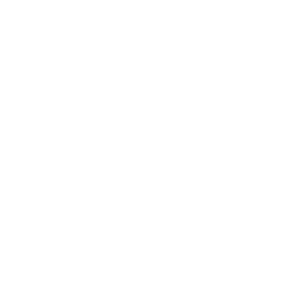
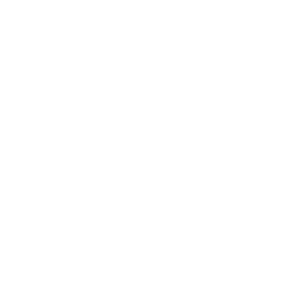
This talk delves into the revolutionary potential of AI agents, powered by generative AI and large language models (LLMs), in transforming ETL (Extract, Transform, Load) and ELT (Extract, Load, Transform) processes.
With an emphasis on automating code generation, streamlining workflows, and enhancing data quality, this session explores how AI-driven solutions are reshaping the landscape of data engineering. Attendees will learn how these intelligent agents can reduce manual coding, eliminate errors, improve operational efficiency, and meet compliance requirements, all while accelerating development timelines.
We will also cover key use cases where AI agents facilitate real-time data transformation, ensure data governance, and promote seamless deployment across cloud environments, giving businesses a competitive edge in today's data-driven world.

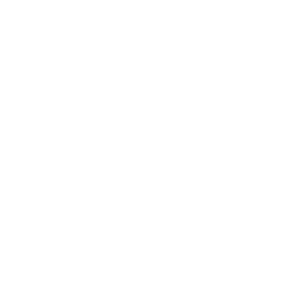
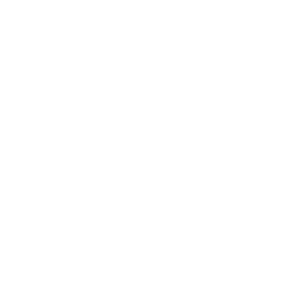
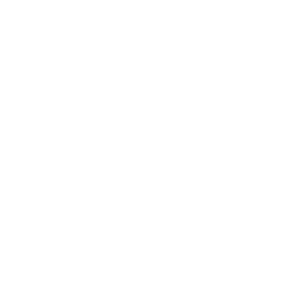
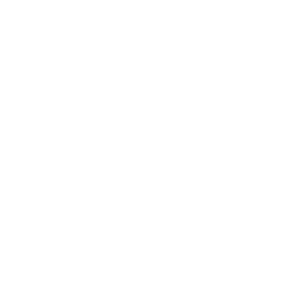
In today’s rush to adopt AI, many organizations overlook a critical truth: value doesn’t come from AI alone—it comes from the powerful combination of software engineering, data engineering, and AI/ML engineering. In this fast-paced, 15-minute talk, Nisha Paliwal draws on 25+ years of experience in banking and tech to unpack the "Trifacta" that fuels real transformation.
From legacy systems to self-learning platforms, she’ll share stories, stats, and insights on how this triad—when integrated—enables banks to move faster, deliver smarter experiences, and generate measurable impact.
Whether you're a technologist, leader, or change agent, you’ll walk away with a fresh lens on cross-functional collaboration, and practical ways to break silos, build trust, and unlock innovation at scale.

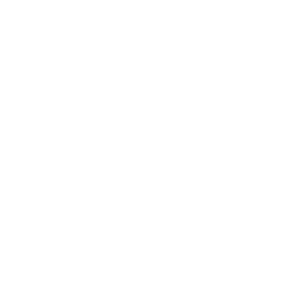
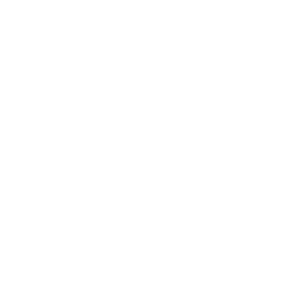
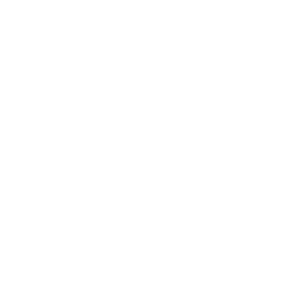
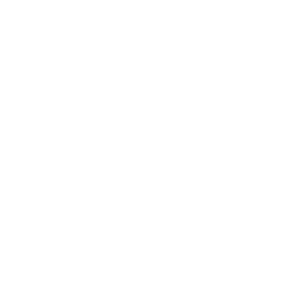
Two very big things happened in recent years that completely transformed the data landscape:
- Pre-trained AI models: These models have democratized AI, enabling software engineers to integrate advanced capabilities into applications with simple API calls and without extensive machine learning expertise.
- Lakehouses: Open formats over object storage bring together the flexibility of data lakes and the data management strengths of data warehouses, offering a more streamlined and scalable approach to data management.
And yet, most data platforms remain difficult to digest for traditional software developers.
This talk introduces "Data as Software," a practical approach to data engineering and AI that leverages the lakehouse architecture to simplify data platforms for developers. By leveraging serverless functions as a runtime and Git-based workflows in the data catalog, we can build systems that makes it exponentially simpler for data developers to apply familiar software engineering concepts to data, such as modular, reusable code, automated testing (TDD), continuous integration (CI/CD), and version control.

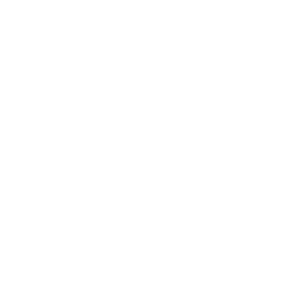
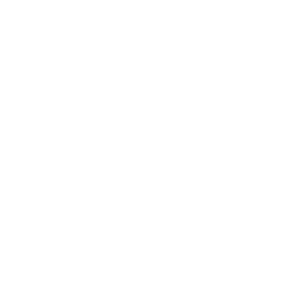
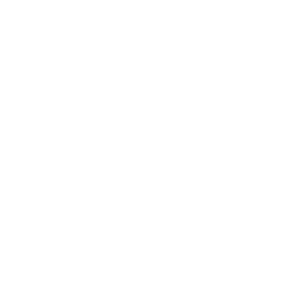
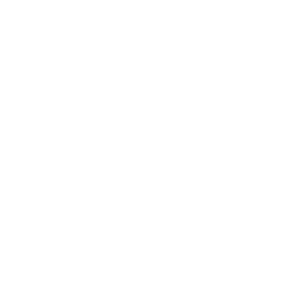
Lorem Ipsum Dolor Sit Amet
Zoom went from a meeting platform to a household name during the COVID-19 pandemic. That kind of attention and usage required significant storage and processing to keep up. In fact, Zoom had to scale their data lakehouse to 100TB/day while meeting GDPR requirements.
Join this session to learn how Zoom built its lakehouse around Amazon Managed Streaming for Kafka (AmazonMSK), Amazon EMR clusters running Apache Spark™ Structured Streaming jobs (for optimized parallel processing of 150 million Kafka messages every 5 minutes) and Apache Hudi on Amazon S3 (for flexible, cost-efficient storage). Raj will talk through the lakehouse architecture decisions, data modelling and data layering, the medallion architecture for data engineering, and how Zoom leverages various open table formations, including Apache Hudi™, Apache Iceberg™ and Delta Lake.

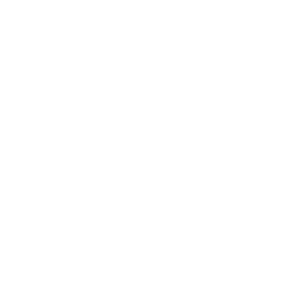
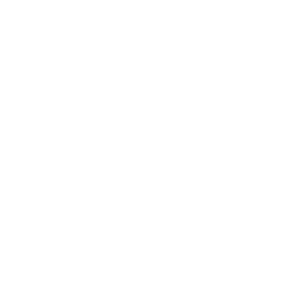
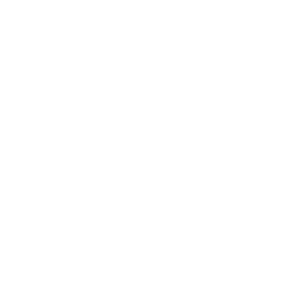
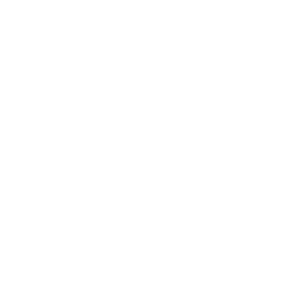
Apache Iceberg™ has become a popular table format for building data lakehouses, enabling multi-engine interoperability. This presentation explains how Google BigQuery leverages Google's planet-scale infrastructure to enhance Iceberg, delivering unparalleled performance, scalability, and resilience.
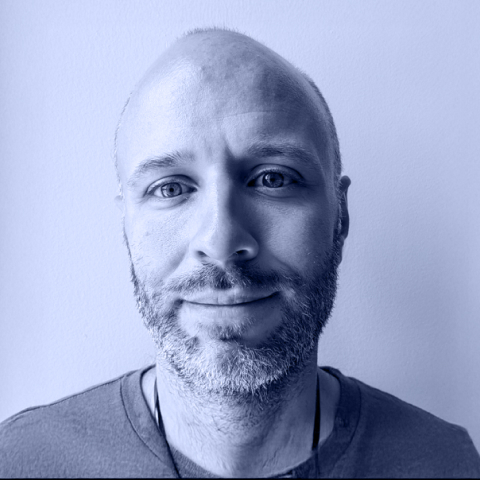
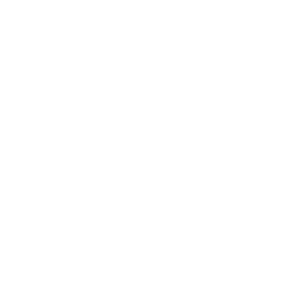
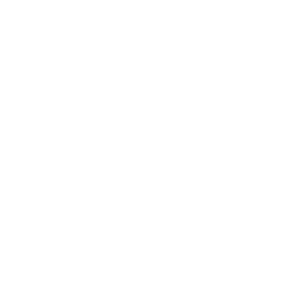
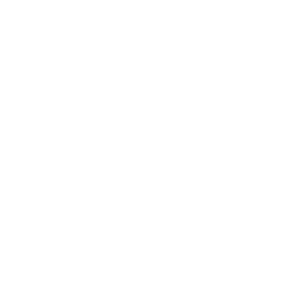
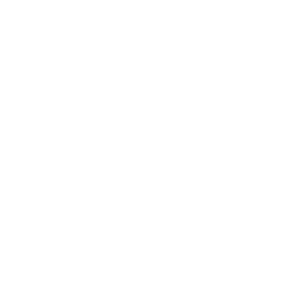
Presto (https://prestodb.io/) is a popular open source SQL query engine for high performance analytics in the Open Data Lakehouse. Originally developed at Meta, Presto has been adopted by some of the largest data-driven companies in the world including Uber, ByteDance, Alibaba and Bolt. Today it’s available to run on your own or through managed services such as IBM watsonx.data and AWS Athena.
Presto is fast, reliable and efficient at scale. The latest innovation in Presto is a state of the art C++ native query execution engine that replaces the old Java execution engine. Presto C++ is built using Velox, which is another Meta open source project for common runtime primitives across query engines. Deployments with the new Presto native engine show massive price performance improvements with fleet sizes shrinking to almost 1/3rd of their Java cluster counterparts, leading to enormous cost savings.
The Presto Native engine project began in 2020 and since then, it has matured into production use at Meta, Uber, and in IBM watsonx.data. This talk gives an in-depth look at this journey, covering:
- Introduction to Prestissimo/Velox architecture
- Production experiences and learnings from Meta
- Benchmarking results from TPC-DS workloads
- New lakehouse capabilities enabled by the native engine
Beyond the product features, we will highlight how the open source community shaped this innovation and the benefits of building technology like this openly across many companies.

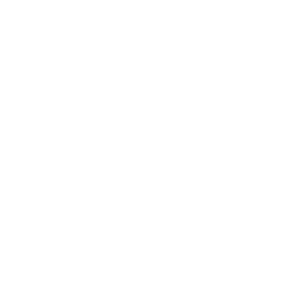

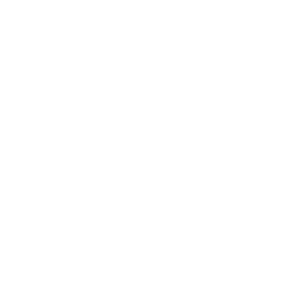
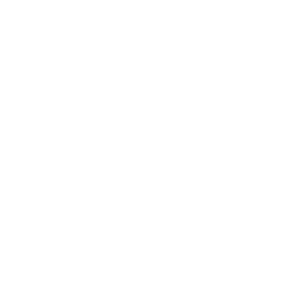
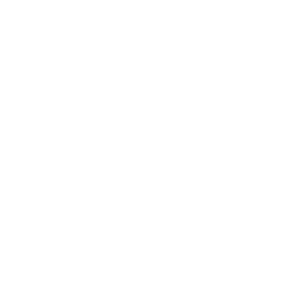
Data engineering teams often struggle to balance speed with stability, creating friction between innovation and reliability. This talk explores how to strategically adapt software engineering best practices specifically for data environments, addressing unique challenges like unpredictable data quality and complex dependencies. Through practical examples and a detailed case study, we'll demonstrate how properly implemented testing, versioning, observability, and incremental deployment patterns enable data teams to move quickly without sacrificing stability. Attendees will leave with a concrete roadmap for implementing these practices in their organizations, allowing their teams to build and ship with both speed and confidence.

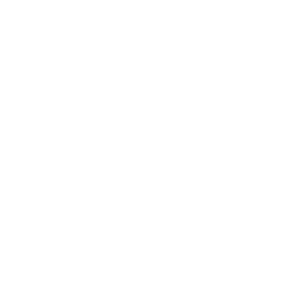
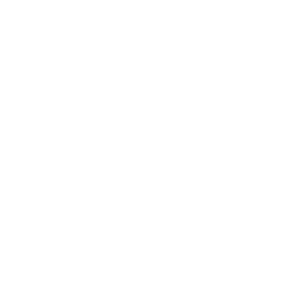
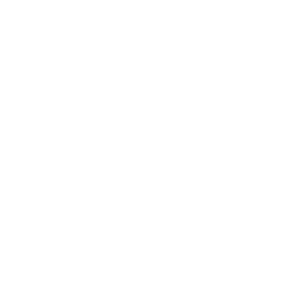
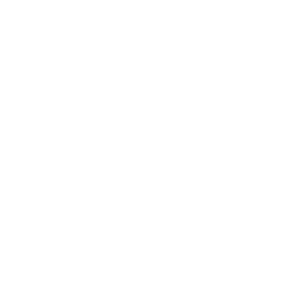
In this talk, Amaresh Bingumalla shares how his team utilized Apache Hudi to enhance data querying on blob storages such as S3. He describes how Hudi helped them cut ETL time and costs, enabling efficient querying without taxing production RDS instances. He also talks about how they leveraged open-source tools such as Apache Hudi™, Apache Spark™, and Apache Kafka™ to build near real-time data pipelines. He explains how they improved their ML and analytics workflows by using some of the key Hudi features such as ACID compliance, time travel queries, and incremental reads, and paired with Datahub, how they boosted data discoverability for downstream systems.

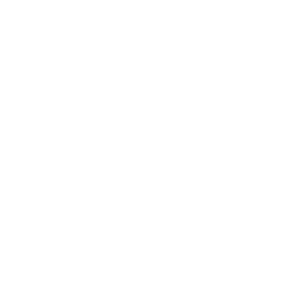
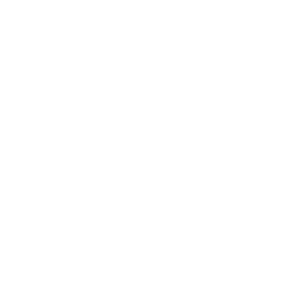
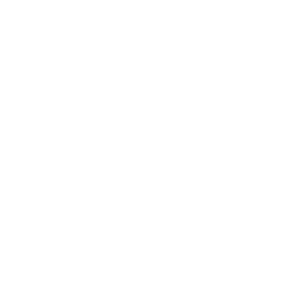
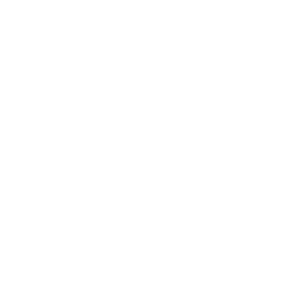
For decades, ODBC/JDBC have been the standard for row-oriented database access. However, modern OLAP systems tend instead to be column-oriented for performance - leading to significant conversion costs when requesting data from database systems. This is where Arrow Database Connectivity comes in!
ADBC is similar to ODBC/JDBC in that it defines a single API which is implemented by drivers to provide access to different databases. However, ADBC's API is defined in terms of the Apache Arrow in-memory columnar format. Applications can code to this standard API much like they would for ODBC or JDBC, but fetch result sets in the Arrow format, avoiding transposition and conversion costs if possible.
This talk will cover goals, use-cases, and examples of using ADBC to communicate with different Data APIs (such as Snowflake, Flight SQL or Postgres) with Arrow-native in-memory data.

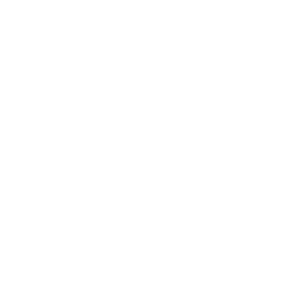
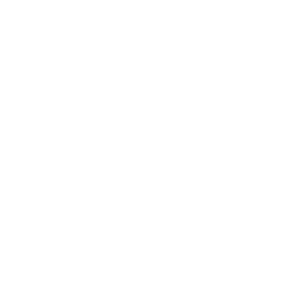
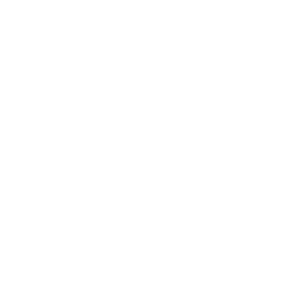
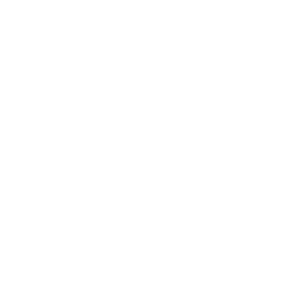
AI/ML systems require realtime information derived from many data sources. This context is needed to create prompts and features. Most successful AI models require rich context from a vast number of sources.
To power this, engineers need to manually split their logic and place it in various data processing “paradigms” - stream processing, batch processing, embedding generation and inference services.
Today practitioners need to spend tremendous effort to stitch together disparate technologies to power for *each* piece of context.
While at Airbnb, we created a system to automate the data and systems engineering required to power AI models both for training / fine-tuning and for online inference.
It is deployed in critical ML pathways and actively developed by Stripe, Uber, OpenAI and Roku (in addition to Airbnb).
In this talk I will go over use cases, the Chronon project overview, and future directions.

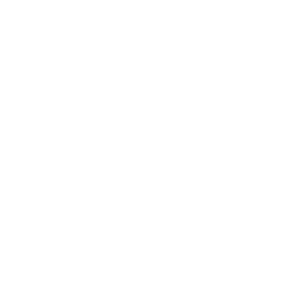
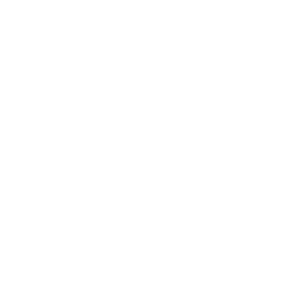
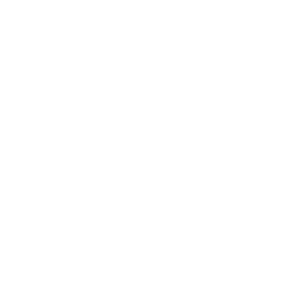
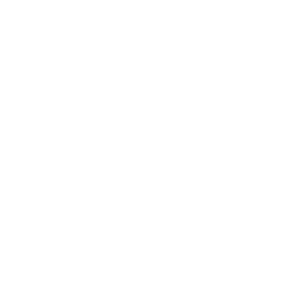
Customer-facing analytics is your competitive advantage, but ensuring high performance and scalability often comes at the cost of data governance and increased data silos. The open data lakehouse offers a solution—but how do you power low-latency, high-concurrency queries at scale while maintaining an open architecture?
In this talk, we’ll dive into the core query engine innovations that make customer-facing analytics on an open lakehouse possible. We’ll cover:
- Key challenges of customer-facing analytics at scale
- Query engine essentials for achieving fast, concurrent queries without sacrificing governance
- Real-world case studies, including how industry leaders like TRM Labs are moving their customer-facing workloads to the open lakehouse
Join us to explore how you can unlock the full potential of customer-facing analytics—without compromising on governance, flexibility, or cost efficiency.

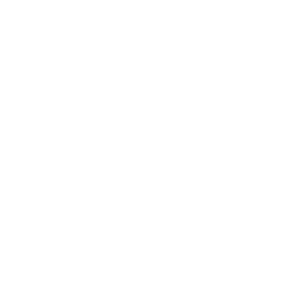
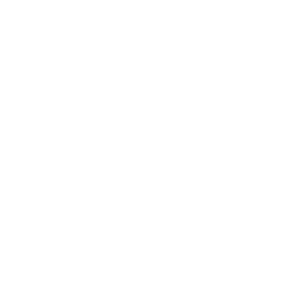
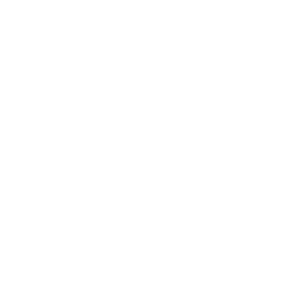
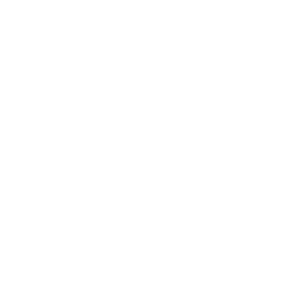
At Twilio, our data mesh enables data democratization by allowing domains to share and access data through a central analytics platform without duplicating datasets—and vice versa. Using AWS Glue and Lake Formation, only metadata is shared across AWS accounts, making the implementation efficient with low overhead while ensuring data remains consistent, secure, and always up to date. This approach supports scalable, governed, and seamless data collaboration across the organization.

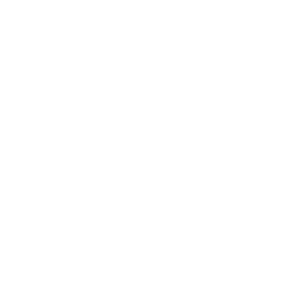
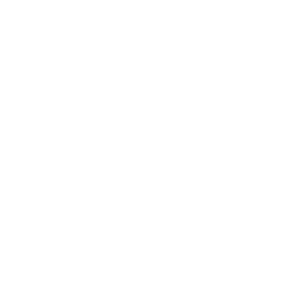
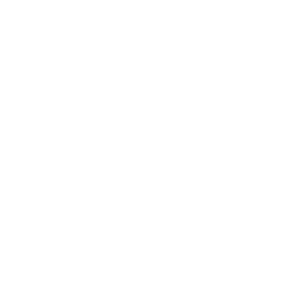
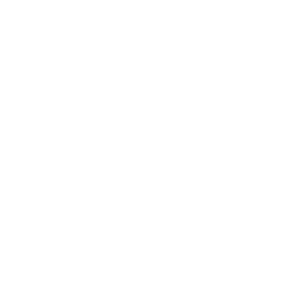